Deep Learning-Based Localization Approach for Autonomous Robots in the RobotAtFactory 4.0 Competition
Deep Learning-Based Localization Approach for Autonomous Robots in the RobotAtFactory 4.0 Competition
Summary
Accurate localization in autonomous robots enables effective decision-making within their operating environment. Various methods have been developed to address this challenge, encompassing traditional techniques, fiducial marker utilization, and machine learning approaches. This work proposes a deep-learning solution employing Convolutional Neural Networks (CNN) to tackle the localization problem, specifically in the context of the RobotAtFactory 4.0 competition. The proposed approach leverages transfer learning from the pre-trained VGG16 model to capitalize on its existing knowledge. To validate the effectiveness of the approach, a simulated scenario was employed. The experimental results demonstrated an error within the millimeter scale and rapid response times in milliseconds. Notably, the presented approach offers several advantages, including a consistent model size regardless of the number of training images utilized and the elimination of the need to know the absolute positions of the fiducial markers.
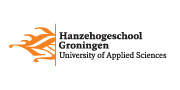
Organisation | Hanze |
Date | 2024-02-03 |
Type | Conference object |
DOI | 10.1007/978-3-031-53036-4_13 |
Language | English |