Optimizing a machine learning design of dielectric properties in lead-free piezoelectric ceramics
Optimizing a machine learning design of dielectric properties in lead-free piezoelectric ceramics
Samenvatting
Designing lead-free piezoelectric ceramics with tailored electrical properties remains a critical challenge for various applications. In this paper we present a novel methodology integrating Machine Learning (ML) and optimization procedures to fine-tune electrical properties in lead-free (1-x) Na0.5 Bi0.5 TiO3 - x CaTiO3 piezoelectric ceramics. A comprehensive dataset of dielectric measurements serves as the foundation for training ML models that accurately predict the permittivity (′) and dielectric loss (tan ) as functions of Ca2+ concentration (x % Ca), temperature and frequency. Two ML techniques are evaluated: random forest regression, and Multi-Layer Perceptron neural network Regression (MLPR). The MLPR model exhibited a superior regression performance, achieving a correlation coefficient of 0.931 and a root mean squared error of 0.029. The MLPR was then optimized by the Non-dominated Sorting Genetic Algorithm II (NSGA-II) to maximizes ′ while minimizes tan . Within the NSGA-II framework, the optimal values were found at the Pareto curve knee, corresponding to a frequency, temperature, and x % Ca of 609.739 kHz, 398.15 K, and 6.10, respectively, resulting in ′ equal to 857.87 and tan equal to 0.0120. This approach demonstrates the effectiveness of combining ML and optimization for designing the electrical properties of piezoelectric ceramics, paving the way for more efficient and targeted material development.
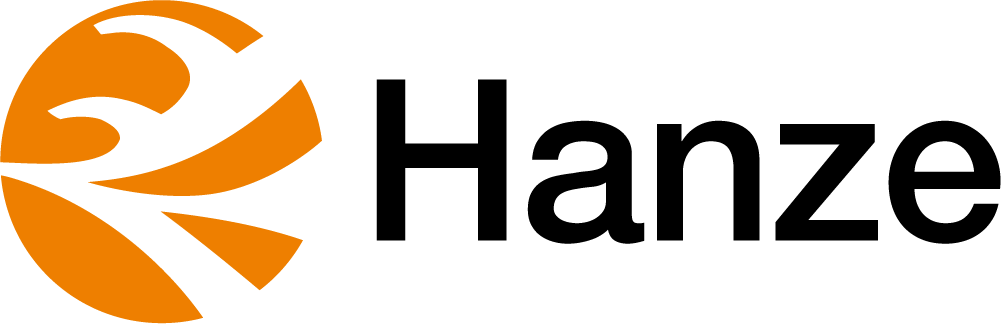
Organisatie | Hanze |
Gepubliceerd in | Materials & Design Elsevier, Vol. 243 |
Datum | 2024-07 |
Type | Artikel |
DOI | 10.1016/j.matdes.2024.113053 |
Taal | Engels |