Online extreme learning on fixed-point sensor networks
Online extreme learning on fixed-point sensor networks
Samenvatting
Anomaly detection is a key factor in the processing of large amounts of sensor data from Wireless Sensor Networks (WSN). Efficient anomaly detection algorithms can be devised performing online node-local computations and reducing communication overhead, thus improving the use of the limited hardware resources. This work introduces a fixed-point embedded implementation of Online Sequential Extreme Learning Machine (OS-ELM), an online learning algorithm for Single Layer Feed forward Neural Networks (SLFN). To overcome the stability issues introduced by the fixed precision, we apply correction mechanisms previously proposed for Recursive Least Squares (RLS). The proposed implementation is tested extensively with generated and real-world datasets, and compared with RLS, Linear Least Squares Estimation, and a rule-based method as benchmarks. The methods are evaluated on the prediction accuracy and on the detection of anomalies. The experimental results demonstrate that fixed-point OS-ELM can be successfully implemented on resource-limited embedded systems, with guarantees of numerical stability. Furthermore, the detection accuracy of fixed-point OS-ELM shows better generalization properties in comparison with, for instance, fixed-point RLS. © 2013 IEEE.
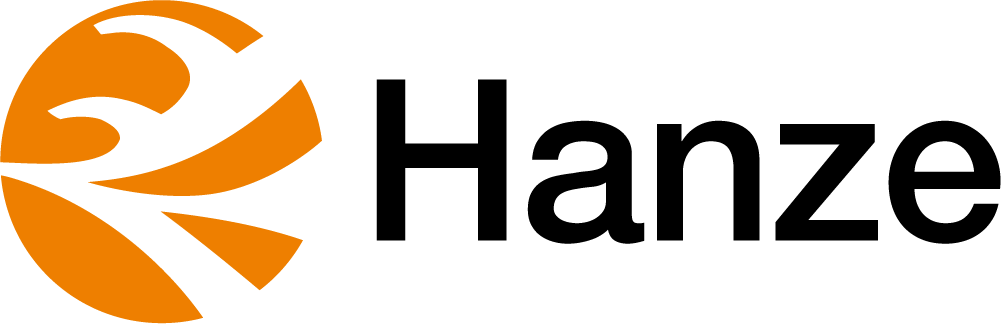
Organisatie | Hanze |
Datum | 2013-12-01 |
Type | Conferentiebijdrage |
DOI | 10.1109/icdmw.2013.74 |
Taal | Engels |