Automatic crack classification and segmentation on masonry surfaces using convolutional neural networks and transfer learning
Automatic crack classification and segmentation on masonry surfaces using convolutional neural networks and transfer learning
Samenvatting
Masonry structures represent the highest proportion of building stock worldwide. Currently, the structural condition of such structures is predominantly manually inspected which is a laborious, costly and subjective process. With developments in computer vision, there is an opportunity to use digital images to automate the visual inspection process. The aim of this study is to examine deep learning techniques for crack detection on images from masonry walls. A dataset with photos from masonry structures is produced containing complex backgrounds and various crack types and sizes. Different deep learning networks are considered and by leveraging the effect of transfer learning crack detection on masonry surfaces is performed on patch level with 95.3% accuracy and on pixel level with 79.6% F1 score. This is the first implementation of deep learning for pixel-level crack segmentation on masonry surfaces. Codes, data and networks relevant to the herein study are available in: github.com/dimitrisdais/crack_detection_CNN_masonry.
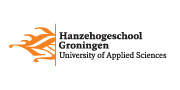
Organisatie | Hanze |
Gepubliceerd in | Automation in Construction Elsevier, Vol. 125 |
Datum | 2021-05 |
Type | Artikel |
DOI | 10.1016/j.autcon.2021.103606 |
Taal | Engels |