Machine Learning Method and Visible Light-Based Sensors for Multiinterface Level Measurement
Machine Learning Method and Visible Light-Based Sensors for Multiinterface Level Measurement
Samenvatting
In this work, a feasible and low-cost approach is proposed for level measurement in multiphase systems inside tanks used for petroleum-derived oil production. The developed level sensor system consisted of light-emitting diodes (LEDs), light-dependent resistor (LDR), and a low-cost microprocessor. Two different types of oil were tested: AW460 and AW68. Linear regression (LR) was applied for 11 scenarios and showed a direct correlation between the level of oil and the sensor’s output. The measurement with AW460 oil presented a perfect linear behavior, while for AW68, a higher standard deviation was obtained justifying the occurrence of the nonlinearity in several scenarios. In order to overcome the nonlinear effect, two machine learning (ML) techniques were tested: K-nearest neighbors regression (KNNR) and multilayer perceptron (MLP) neural network regression. The highest correlation coefficient ( R2 ) and the lowest root mean squared error (RMSE) were obtained for AW68 with MLP. Therefore, MLP was used for regression (level prediction for water, oil, and emulsion) as well as classification (identify the type of oil in the reservoir) simultaneously. The suggested network exhibited a high accuracy for oil identification (99.801%) and improved linear performance in regression ( R2 = 0.9989 and RMSE = 0.065).
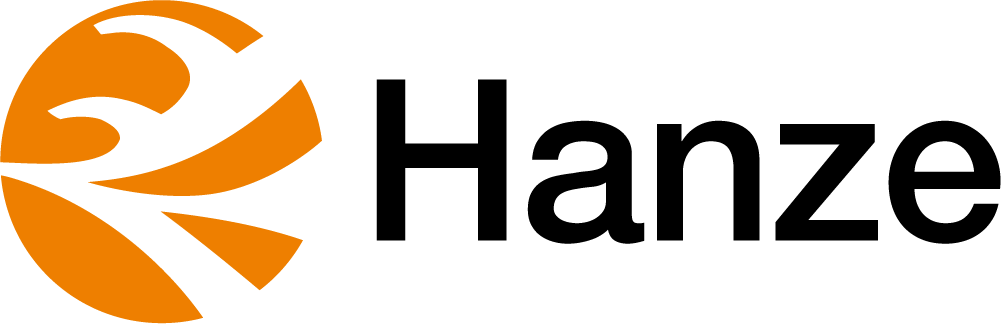
Organisatie | Hanze |
Gepubliceerd in | IEEE sensors journal IEEE, Vol. 23, Uitgave: 14, Pagina's: 16393-16401 |
Datum | 2023-06-08 |
Type | Artikel |
DOI | 10.1109/JSEN.2023.3282026 |
Taal | Engels |